The Rise of AI and the Need for Efficient Thermal Management
Team Expert Thermal2024-08-22T13:38:54+00:00Unleashing the Power of AI
The rapid growth of Artificial Intelligence (AI) relies on specialized AI chips, which present unique thermal management challenges. Efficient AI chip cooling solutions are critical for handling the intensive processing tasks such as deep learning, data analysis, and natural language processing. Expert Thermal offers cutting-edge thermal management solutions designed specifically for AI-powered systems, ensuring optimal performance and energy efficiency.
The world of AI chips for optimized performance and thermal efficiency is a diverse landscape, boasting a variety of options tailored for specific purposes:
- Graphics Processing Units (GPUs): Initially designed to accelerate graphics processing and image manipulation, GPUs have become a cornerstone of AI development due to their parallel processing prowess. This allows AI systems to leverage multiple GPUs simultaneously for significant performance gains.
- Field-Programmable Gate Arrays (FPGAs): These highly customizable chips offer a unique advantage – they can be reprogrammed at the hardware level. This flexibility, however, comes at the cost of requiring specialized programming expertise.
- Neural Processing Units (NPUs): Custom-built for the demands of deep learning and neural networks, NPUs excel at handling massive datasets and complex workloads. Their specialized architecture empowers them to tackle tasks like image recognition and natural language processing with remarkable speed and efficiency.
- Application-Specific Integrated Circuits (ASICs): These champions of performance are designed and built from the ground up for a single, specific AI application. While they lack the reprogrammability of FPGAs, their focused design translates into unparalleled processing power for their designated AI tasks.
AI Chip Battlefield: A Power Struggle for AI Supremacy
The realm of AI chips is a dynamic battleground, with established players like Nvidia, Intel, and AMD locked in intense competition alongside emerging challengers. Here is a breakdown of the key contenders and their latest offerings, highlighting their strengths and power considerations:
Fig 1: Generative AI Market Share Information (2023)
Nvidia
Blackwell Architecture: Nvidia’s latest innovation boasts significant performance and efficiency improvements. Blackwell improves AI inferencing performance by 30 times and does it with up to 25 times better energy efficiency than Nvidia’s previous generation Hopper architecture chips. Blackwell unifies two dies into one GPU. Blackwell GPUs boast a massive leap in processing power compared to their predecessors. Blackwell boasts the largest chip ever created for a GPU, packing a staggering 104 billion transistors. This significant jump in transistor count is achieved through a dual-chipset design utilized by the Blackwell B100 and B200 GPUs.
This innovative design translates to a substantial performance boost. For example, the B100 packs a whopping 128 billion more transistors than the current H100 GPU, delivering five times the AI performance.
The 3 Blackwell architecture configurations:
- B200 Discrete GPU (up to 1000 watts) delivers 144 petaflops of AI performance in an eight-GPU configuration. This supports x86-based generative AI platforms and supports networking speeds of up to 400 GB/s through Nvidia’s newly announced Quantum-X800 InfiniBand and Spectrum-X800 Ethernet networking switches.
- B100 Discrete GPU (up to 700 watts) delivers 112 petaflops of AI performance, designed for upgrading existing data center infrastructure.
- (2 GPU and 1 CPU) (up to 2700 watts) delivers 40 petaflops of performance. It combines two NVIDIA B200 Tensor Core GPUs with Grace CPU 144 ARM Neoverse V2 cores over 900 GB/s ultra-low-power NVLink chip-to-chip interconnect.
Fig 2: GB200 Grace Blackwell Superchip
NVIDIA’s 2 notable GPU platforms: NVIDIA DGX and NVIDIA HGX
NVIDIA DGX systems are high-performance computing platforms specifically designed for tackling demanding artificial intelligence (AI) workloads. They pack multiple high-powered NVIDIA GPUs interconnected for fast data transfer and come pre-loaded with deep learning software tools like CUDA and TensorRT. This lets researchers and data scientists develop and deploy AI models efficiently. DGX systems boast exceptional performance, delivering high petaFLOPS (PFLOPS) for training large and complex AI models, making them a powerful solution for innovative AI endeavors.
- NVIDIA DGX B200: A Blackwell platform that combines 8x NVIDIA Blackwell GPUs with 1,440GB of GPU to deliver 72 petaFLOPS training and 144 petaFLOPS inference. The is a powerhouse in terms of performance and, correspondingly, power consumption. Operating the DGX B200 requires approximately 14.3 kW of power. In a typical data center configuration, this translates to around 60 kW of rack power when factoring in additional system components and potential peak usage scenarios. Operating temperature of 5-30oC
- NVIDIA DGX™GB200 NVL72: A Blackwell platform that connects 36 Grace CPUs and 72 Blackwell GPUs in a rack-scale design. The GB200 NVL72 is a liquid-cooled, rack-scale solution that serves as the foundation of forthcoming new Nvidia SuperPOD AI Supercomputers that will enable large, trillion-parameter-scale AI models. Amazon Web Services, Google Cloud and Oracle Cloud Infrastructure will make GB200 NVL72 instances available on the Nvidia DGX CloudAI supercomputer cloud service. NVIDIA did not announce any power consumption figures, industry estimates place the power requirement at approximately 50 kW per rack, GB200 NVL72’s performance and energy efficiency and the fact that the system offers 1440 petaflops and 720 petaflops of performance for training – nearly one exaflop – in one rack. For example, organizations can train a GPT 1.8 trillion parameter model in 90 days using 8,000 Hopper GPUs while using 15 MW of energy. With Blackwell, organizations could train the same model in the same amount of time using just 2,000 Blackwell GPUs, while using only 4 MW of power
Fig 3: GB200 NVL72 Architecture
NVIDIA HGX is a hardware specification designed for flexibility and customization in GPU platforms. “HGX” stands for “Hyperscale Graphics eXtension.” This modular hardware design establishes standards for hardware interfaces and interconnects, allowing partners to create custom GPU systems suited to their specific needs. It includes GPUs, interconnect technologies, and other essential components that can be integrated with various hardware platforms. The modular nature of HGX allows it to cater to diverse scales and applications, ranging from data centers to supercomputers
Nvidia’s AI Roadmap: A Look Ahead
- 2024: Nvidia is on track to phase out older AI GPUs like the H100 in favor of the new B100 series. This transition is expected to be completed by the end of the year (Q3 or Q4). Additionally, the GB200 platform will be introduced, combining the powerful Grace Hopper CPU with the B200 AI GPU for even greater processing capabilities.
- 2025: Get ready for the launch of the Blackwell Ultra GPU, Nvidia’s next step in AI processing power. This year will also see the introduction of the Spectrum Ultra X800 Ethernet Switch, further solidifying Nvidia’s push into high-performance AI networking solutions.
- 2026 and Beyond: The roadmap extends into the future, with plans for the Rubin GPU, Vera CPU, and the next generation of networking switches (NVLink 6, CX9 SuperNIC, and X1600) scheduled for release in 2026. The roadmap culminates in 2027 with the unveiling of the Rubin Ultra GPU, hinting at even more powerful options to come.
Fig 4: Nvidia’s Roadmap
Intel
- Gaudi 3 AI Accelerator (GPU): Designed for AI training and inferencing, it offers: 4x more AI compute power and 1.5x more memory bandwidth than its predecessor, Gaudi 2. It is projected to deliver 50% faster training/inferencing and 40% better inferencing power efficiency compared to Nvidia’s H100 GPU. Total Device Power (TDP) of 900 Watts with passive cooling and 1.2kW with liquid cooling. With temperature range from 17-35oC
- Xeon 6 Processor Family (CPU): Sierra Forest (“E-core”) is designed for hyperscalers and cloud service providers who want power efficiency and performance. Sierra Forest focuses on balancing performance with energy efficiency, potentially becoming the industry leader in core count (144 cores-6700, 288 cores-6900). 330-205 Watts TDP. Granite Rapids (“P-core”) prioritizes high performance, aiming for 2-3x better performance for AI workloads than its predecessor and focuses on high performance. 350-380 Watts TDP
AMD
- Instinct MI300X Series GPUs: Launched in December, these GPUs target AI training and inferencing. 750 W AMD claims MI300 outperforms Nvidia’s H100 and upcoming H200 GPUs.
- Turin (CPU): Their next-generation server processor based on the Zen 5 core architecture is planned for release. 192 cores with 500W TDP
Other Players
- Ampere One (CPU): AmpereOne Arm-compatible server processors offer impressive performance per rack, exceeding AMD’s Genoa, and Intel’s Sapphire Rapids chips. 192 cores with 350W TDP. AmpereOne can run 7,296 virtual machines (VMs) per rack, which is 2.9 times more than AMD’s Genoa and 4.3 times more than Intel’s Sapphire Rapids chip. As for generative AI, AmpereOne can produce 2.3 times more frames or images per second than AMD’s Genoa chip
AWS: This year, AWS will introduce:
- Graviton4 (CPU) for general-purpose workloads. 205 W TDP
- Trainium2 for AI training (4x the computer power and 3x the memory of its predecessor).
- Inferentia2, their second-generation AI inferencing chip, is already available.
Google Cloud: A pioneer in custom AI chips, Google Cloud offers Tensor Processing Units (TPUs) for training and inferencing. They recently launched their fifth-generation TPU, the Cloud TPU v5p, delivering 2.8x faster model training compared to the previous generation. Google Cloud also unveiled its first Arm-based CPUs, the Google Axion Processors. Axion will be used in many Google Cloud services, including Google Compute Engine, Google Kubernetes Engine, Dataproc, Dataflow and Cloud Batch.
Power Considerations: Compared to other data center uses, powering AI and high-performance computing (HPC) requires 5-10 times more energy. This surge is due to the increasing adoption of advanced AI technologies and denser server deployments within data centers. Energy consumption is a growing concern. A 2022 report by the International Energy Agency estimates that U.S. data centers already account for over 4% of the nation’s electricity use, and this is projected to climb to 6% by 2026.
As data storage and processing demands continue to rise, so will energy consumption. Traditional data centers typically require 8-15 kW per rack, but AI-ready racks equipped with powerful GPUs can demand a staggering 40-60 kW.
Nvidia’s new Blackwell chips, for example, necessitate robust cooling due to their high-power requirements. In dense data center configurations, these chips could push overall energy needs from 60 kW per rack to a staggering 120 kW. In the next few years, peak power density within data centers could even reach 150 kW per rack.
The amount of electricity a data center devours depends on its size and the efficiency of its equipment. Smaller facilities, typically housing 500-2,000 servers across 5,000-20,000 square feet, typically consume 1-5 MW of power. On the other hand, massive hyperscale data centers, sprawling across 100,000 square feet or even millions, and accommodating tens of thousands of servers, can guzzle a staggering 20 MW to over 100 MW.
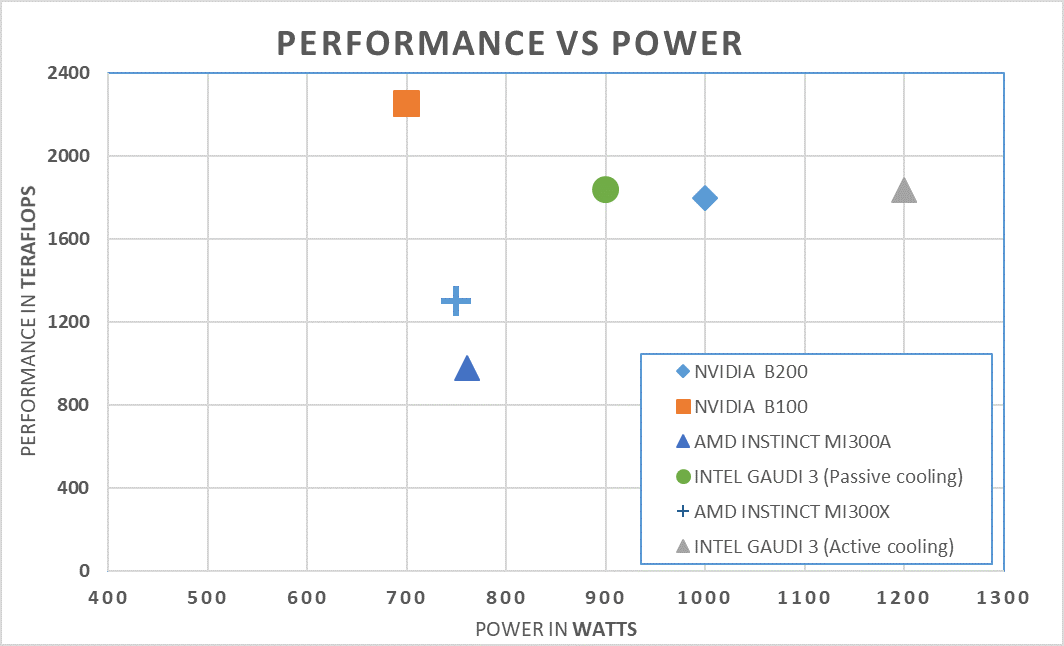
Fig 5: AI GPU comparison chart
These power-hungry giants are filled with constantly running systems that collectively consume a significant amount of energy. This power is typically divided into two categories: that used by the IT equipment itself (servers, storage, networks) and that used by the supporting infrastructure (cooling, power conditioning).
Fig 6: Data center electricity usage evaluation
Fig 7: Generative AI power demand for AI Training and Inference
Here’s a surprising fact: Data center cooling systems alone can account for up to 50% of a facility’s total power consumption. This highlights the need for efficient cooling solutions to minimize energy waste.
Fig 8: Breakdown of Energy Consumption by Different Components of a Data Center
With over 10,900 data centers worldwide as of December 2023, and over 45% concentrated in the US, the global demand for data storage and processing is immense. This translates to a significant energy footprint. McKinsey estimates power needs of U.S. data centers will jump from 2022’s 17 GW to 35 GW by 2030.
We can very well imagine that the need for power to run the data centers will continue to grow substantially. Expert Thermal addresses this challenge by providing energy-efficient cooling solutions tailored for AI-powered data centers, minimizing energy waste while ensuring peak performance of AI chips and high-performance computing systems. By investing in innovative technologies and sustainable practices, data centers can ensure their infrastructure will not only meet this growing demand but also minimize environmental effects by reducing power consumption.
Thermal Management of AI powered systems:
The Evolving Landscape of Data Center Cooling: Data centers have historically relied on air cooling for their workloads. However, the surge in AI and high-performance computing (HPC) applications presents a new challenge: significantly higher power densities. These advanced workflows often require specialized cooling solutions like direct liquid cooling (DLC), air-assisted liquid cooling (AALC), or rear-door heat exchangers.
Understanding Your Needs: It’s crucial to recognize that cooling needs vary across diverse AI and HPC workloads. Not every server will necessitate advanced cooling. For instance, inferencing deployments typically have lower power requirements than training and may be adequately cooled with traditional air methods. Similarly, machine learning demands less power compared to deep learning and generative AI, which require substantial resources due to their complexity.
The Importance of Expertise and Customization: Selecting the optimal cooling solution requires a nuanced understanding of your specific requirements. A “one-size-fits-all” approach simply will not suffice. Partnering with a data center cooling expert allows for the development of a customized strategy that aligns with your unique needs. This expertise becomes even more critical when considering the diverse cooling demands within AI and HPC workflows.
Liquid Cooling: The rapid advancements in AI and HPC necessitate a change in basic assumptions in Expert Thermal’s innovative data center cooling strategies. Traditional air-cooling struggles to effectively dissipate the heat generated by high-power density chips, potentially leading to overheating, reduced performance, and increased operational costs. Liquid cooling emerges as a game-changer, offering exceptional heat dissipation capabilities ideal for modern data centers housing high-wattage processors.
At Expert Thermal, we specialize in developing sustainable liquid cooling solutions that not only reduce energy consumption but also extend the lifespan of high-performance computing equipment.
Benefits of Liquid Cooling
Liquid cooling offers a multitude of advantages over traditional cooling methods, including:
- Reduced Energy Consumption: Liquid cooling cuts your data center’s energy footprint by requiring significantly less power compared to traditional air-cooling methods. This translates to cost savings and a more sustainable operation.
- Sustained Peak Performance: These systems excel at maintaining optimal performance for your hardware, even as you scale to higher processing power. Achieve more without sacrificing efficiency.
- Extended Equipment Lifespan: By minimizing the risk of overheating, liquid cooling solutions keep your equipment running cooler and longer, maximizing your return on investment.
- Enhanced Safety: Water-based and non-conductive fluids create a safer environment for both personnel working around the equipment and the equipment itself.
- Green by Design: Innovative single-phase direct liquid cooling contributes to a greener data center by reducing carbon emissions, saving energy, and lowering noise pollution. This technology minimizes reliance on traditional air-cooling systems, shrinking your overall environmental impact.
Expert Thermal: Your Trusted Partner for Data Center Cooling Innovation
We take the guesswork out of managing your data center’s thermal challenges. Our comprehensive portfolio of cutting-edge products, systems, and services is designed to meet the evolving needs of AI and HPC implementations. From Cooling Distribution Units (CDUs) to Cold Plates, we offer a holistic solution to ensure optimal performance and energy efficiency.
Greenhouse Gas Emissions: The looming shadow for the data driven world
The ever-growing demand for artificial intelligence (AI) and large language models (LLMs) like GPT-3 comes with a hidden cost: a significant carbon footprint. Studies have shown that training these models can be incredibly energy-intensive, releasing substantial amounts of greenhouse gas (GHG) emissions.
- Training a single AI model can emit over 626,000 pounds (about 283948.59 kg) of CO2, equivalent to the lifetime emissions of five cars [University of Massachusetts Amherst, 2019].
- The development of GPT-3, for instance, generated over 550 tons of CO2, the same as 550 roundtrip flights between New York and San Francisco [Kate Saenko, computer scientist].
- Google’s carbon footprint jumped by 48% from 2019, amounting to 14.3 million tons of carbon dioxide emissions for 2023.
These figures highlight the environmental impact of AI development.
While training is a major culprit, research suggests that inference, the stage where AI models make predictions and answer queries, may be even more energy demanding. This ongoing power consumption further contributes to data center emissions.
According to the International Energy Agency (IEA), data centers and data transmission networks were responsible for 330 million metric tons of CO2 emissions in 2020. This number is projected to rise as global data center power needs increase.
The tech industry is starting to address this challenge. Companies like Google, Meta (formerly Facebook), and Amazon are investing in renewable energy sources and innovative data center designs to reduce their carbon footprint.
- Google aims for 24/7 carbon-free energy by 2030 and has already achieved 100% renewable energy for its data centers.
- Meta’s data centers boast a PUE ratio of 1.08 (2022), indicating efficient energy usage. Their Open Compute Project servers and “vanity-free” design contribute to this efficiency.
- Amazon is committed to achieving net-zero carbon emissions by 2040, powered by 100% renewable energy for its operations and AWS data centers by 2025.
Fig 9: Electricity Consumption and GHG emissions comparison and forecast
From the graph displayed there is a clear trend of rising electricity consumption by data centers. This translates to a potential increase in greenhouse gas (GHG) emissions since a significant portion of the world’s electricity comes from fossil fuels like coal and natural gas, which release CO2 during power generation.
The traditional methods of electricity generation for data centers significantly contribute to GHG emissions. Coal combustion, historically the dominant source, releases far more carbon dioxide than natural gas or petroleum. However, in the United States (2022), despite accounting for 55% of CO2 emissions from the power sector, coal only generated 20% of electricity. Natural gas emerged as the leading source, responsible for 39% of electricity generation, while petroleum use remained minimal (less than 1%). There’s a shift towards cleaner energy sources. The remaining 40% of electricity generation in the US (2022) came from non-fossil fuel sources: nuclear (19%) and renewable energy (21%), including hydroelectricity, biomass, wind, and solar. These non-emitting sources offer a path towards a more sustainable future for data centers.
Dubai’s Moro Hub, officially announced as the largest solar-powered data center in the world, reduces approximately 13,806 tons of CO2 emissions annually. The facility follows the standards for green buildings to achieve net-zero emissions by 2050. The training of AI startup Hugging Face’s large language model BLOOM with 176 billion parameters consumed 433 MWh of electricity, resulting in 25 metric tons of CO2 equivalent. It was trained on a French supercomputer run on nuclear energy
While these efforts are commendable, continued innovation and collaboration are essential to minimize the environmental impact of the data-driven world. Exploring artificial intelligence algorithms with lower energy requirements and further optimizing data center efficiency are crucial steps in achieving a sustainable future for AI advancements.
Conclusion
The booming world of AI promises incredible advancements, while it unlocks incredible potential, its energy demands strain sustainability. Addressing this challenge requires innovative solutions that prioritize both performance and environmental responsibility. Let’s embrace AI responsibly.
The ever-growing demand for high-performance computing across various industries creates a critical need for innovative thermal management solutions. Expert Thermal tackles this challenge head-on with a comprehensive suite of innovative thermal solutions. Our advanced systems go beyond traditional air cooling, offering superior heat transfer and energy efficiency. This translates to maximized uptime, extended equipment lifespan, and reduced operational costs. Partner with Expert Thermal and unlock the full potential of your high-performance computing environment.
Reference:
- https://www.cio.com/article/2500767/ahead-of-the-curve-on-advanced-cooling-for-ai-hpc.html
- https://www.motivaircorp.com/news/the-future-of-ai-factories-and-liquid-cooling/
- https://www.constellationr.com/blog-news/insights/nvidia-outlines-roadmap-including-rubin-gpu-platform-new-arm-based-cpu vera#:~:text=Nvidia%20CEO%20Jensen%20Huang%20outlined,has%20a%20one%2Dyear%20rhythm.
- https://www.moomoo.com/community/feed/nvidia-s-product-roadmap-112119997530117
- https://www.ibm.com/think/topics/ai-chip#:~:text=AI%20chips%20are%20logic%20chips,capabilities%20and%20smaller%20energy%20footprints.
- https://www.sunbirddcim.com/blog/data-center-energy-consumption-trends-2024
- https://www.whitecase.com/insight-our-thinking/constructing-low-carbon-economy-data-centers-demands
- https://abcnews.go.com/Business/data-centers-fuel-ai-crypto-threaten-climate-experts/story?id=109342525
- https://carboncredits.com/carbon-countdown-ais-10-billion-rise-in-power-use-explodes-data-center-emission/
- https://www.informationweek.com/sustainability/ai-data-centers-and-energy-use-the-path-to-sustainability
- https://www.datacenterknowledge.com/energy-power-supply/data-center-power-fueling-the-digital-revolution
- https://techhq.com/2024/01/how-the-demands-of-ai-are-impacting-data-centers-and-what-operators-can-do/
- https://www.datacenterknowledge.com/data-center-chips/data-center-chips-in-2024-top-trends-and-releases
- https://www.datacenterfrontier.com/network/article/33038205/how-ai-is-affecting-data-center-networks
- https://www.datacenterfrontier.com/cooling/article/55002575/for-ai-and-hpc-data-center-liquid-cooling-is-now
- Google reveals 48% increase in greenhouse gas emissions from 2019, largely driven by data center energy demands | Tom’s Hardware (tomshardware.com)
- https://www.forbes.com/sites/bethkindig/2024/06/20/ai-power-consumption-rapidly-becoming-mission-critical/
Leave a Reply